⚡一分钟速读:DeepSeek启示录 🌟
🚀 中国AI崛起新势力
-
生成式AI赛道中,中国正快速缩小与美国的差距 -
深度求索(DeepSeek)等推出对标OpenAI的顶尖模型(如R1) -
视频生成等领域已现局部领先 🌐
💡 开源浪潮重塑AI生态
-
MIT许可的DeepSeek-R1开启「开源权重」新范式 -
基础模型层加速商品化 → 应用开发黄金时代到来 -
警惕:开源主导权或成中美价值观博弈新战场 ⚖️
🎯 算法创新>无脑堆算力
-
突破芯片封锁:H800上跑出600万美元低成本模型 -
训练成本下降 ≠ 算力需求减少(智能需求无上限📈) -
资本叙事转向:规模扩张不再是唯一真理
🌍 未来展望
-
地缘影响深远:AI供应链重构进行时 -
开发者利好:高级推理模型触手可及 -
此刻正是创新应用爆发前夜 ✨
Andrew结语
“现在依然是构建AI应用的黄金时代!” 🚀
DeepSeek 启示录:开源、普惠与 AI 的未来图景
朋友们,
本周,DeepSeek 掀起的热潮让很多人看清了几个在我们眼皮底下悄然发生的重要趋势:
-
中国在生成式 AI 领域正奋起直追 —— 这将对 AI 供应链产生深远影响; -
开源权重模型正加速基础模型层的商品化 —— 为应用开发者们创造前所未有的机遇; -
一味追求规模扩张并非 AI 进步的唯一途径 —— 尽管算力相关的话题被炒得火热,但算法创新正迅速降低训练成本。
Dear friends,
This week, the buzz surrounding DeepSeek has crystallized several important trends that have been unfolding right before our eyes:
-
China is catching up in generative AI — with profound implications for the AI supply chain; -
Open weight models are accelerating the commoditization of the foundation-model layer — creating unprecedented opportunities for application builders; -
Scaling up isn’t the only path to AI progress — even though there’s massive hype around compute, algorithmic innovations are rapidly driving down training costs.
DeepSeek-R1:引发关注的卓越模型
大约一周前,来自中国的 DeepSeek 发布了 DeepSeek-R1 —— 一款在各项基准测试中可与 OpenAI 的 o1 媲美的卓越模型。更令人振奋的是,它以开源权重模型的形式推出,并采用了极为宽松的 MIT 许可协议。上周在达沃斯,我接到了不少非技术出身的商业领袖关于它的提问;而就在本周一,市场上还上演了一场“DeepSeek 抛售”:英伟达及多家美国科技公司的股价应声下跌(截至本文撰写时,股价已有所回升)。
About a week ago, a Chinese company called DeepSeek released DeepSeek-R1, a remarkable model whose benchmark performance rivals OpenAI’s o1. Even more exciting, it was launched as an open weight model under a very permissive MIT license. At Davos last week, I received numerous questions about it from non-technical business leaders, and on Monday, the market experienced a “DeepSeek selloff” — with share prices of Nvidia and several other U.S. tech companies plunging (though they have partially recovered as of this writing).
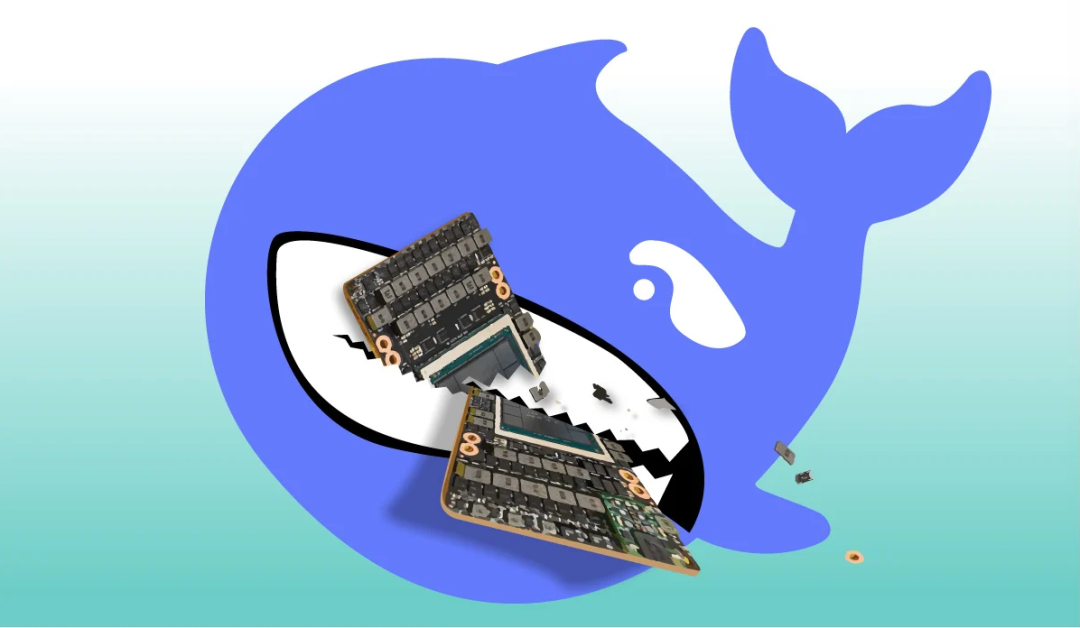
关键启示
1. 中国在生成式 AI 领域正迎头赶上
当 ChatGPT 于 2022 年 11 月横空出世时,美国在生成式 AI 领域明显领先。但刻板印象改变总是缓慢的,直到最近,我还听到不少美国和中国的朋友认为中国还落后。事实上,这一差距在过去两年里已迅速缩小。随着 Qwen(我的团队已使用数月)、Kimi、InternVL 以及 DeepSeek 等一系列优秀模型不断问世,中国显然在奋力追赶,甚至在视频生成等特定领域偶有领先之势。
我非常欣喜地看到 DeepSeek-R1 以开源权重模型形式发布,并附上详尽的技术报告,公开了众多技术细节。相比之下,一些美国公司却借助“人类灭绝”等危言耸听的 AI 威胁论,大力推动监管以扼杀开源。现实已经证明,开源/开源权重模型正成为 AI 供应链中不可或缺的一环,未来将有大量公司采用这些模型。如果美国继续阻碍开源的发展,中国势必会在这一关键环节中占据主导地位,最终许多企业所用的模型将更多反映中国的价值观而非美国的。
1. China is catching up in generative AI. When ChatGPT burst onto the scene in November 2022, the U.S. was clearly ahead. Yet, perceptions change slowly — until recently, many friends in both countries still believed China was lagging behind. In reality, that gap has rapidly narrowed over the past two years. With a stream of impressive models from China such as Qwen (which my team has used for months), Kimi, InternVL, and DeepSeek, China is clearly closing in, even taking the lead at times in areas like video generation.
I’m thrilled that DeepSeek-R1 was released as an open weight model, with a technical report that shares many details. In contrast, a number of U.S. companies have pushed for regulation to stifle open source by hyping up hypothetical AI dangers such as human extinction. It is now clear that open source/open weight models are a key part of the AI supply chain: Many companies will use them. If the U.S. continues to stymie open source, China will come to dominate this part of the supply chain and many businesses will end up using models that reflect China’s values much more than America’s.
2. 开源权重模型正加速基础模型层的商品化
正如我之前所写,大语言模型(LLM)的 token 价格正在快速下降,而开源模型正加速这一趋势,并赋予开发者更多选择。
-
OpenAI o1 价格:每百万输出 token 需 60 美元 -
DeepSeek-R1 价格:仅需 2.19 美元
这一 近 30 倍 的价格差异,让更多人开始关注 AI API 价格下降的趋势。
目前,训练基础模型并通过 API 提供访问权限的商业模式面临严峻挑战。许多公司仍在寻找如何回收巨额训练成本的路径。《AI 的 6000 亿美元问题》一文对此作出了深刻探讨(当然,我依然认为这些基础模型公司在做着卓越的工作,也希望它们取得成功)。相比之下,在基础模型之上构建应用,则有着巨大的商业机会。如今,即便不投资数十亿美元训练模型,企业也可以花费极少的成本来构建 AI 客服、邮件摘要工具、AI 医生、法律文档助手等多种应用。
2. Open weight models are accelerating the commoditization of the foundation-model layer.
As I wrote previously, LLM token prices have been falling rapidly, and open weights have contributed to this trend and given developers more choice.
-
OpenAI’s o1 costs $60 per million output tokens; -
DeepSeek R1 costs $2.19.This nearly 30x difference brought the trend of falling prices to the attention of many people.
The business of training foundation models and selling API access is tough. Many companies in this area are still looking for a path to recouping the massive cost of model training. The article “AI’s $600B Question” lays out the challenge well (but, to be clear, I think the foundation model companies are doing great work, and I hope they succeed). In contrast, building applications on top of foundation models presents many great business opportunities. Now that others have spent billions training such models, you can access these models for mere dollars to build customer service chatbots, email summarizers, AI doctors, legal document assistants, and much more.
3. 盲目追求规模扩张并非 AI 进步的唯一途径
长久以来,业界一直大肆炒作通过扩大模型规模来推动 AI 进步的理念。客观来说,我曾是这一理念的早期支持者。许多公司凭借“更多资本即可(一)扩大规模、(二)可预测地推动改进”的说辞成功筹集了数十亿美元,从而使人们过分关注规模扩张,而忽略了其他多种推动 AI 进步的方式。受美国 AI 芯片禁运政策的部分影响,DeepSeek 团队在众多方面进行了创新和优化,使其模型能够在性能相对较弱的 H800 GPU 上高效运行(而非依赖性能更强的 H100),最终他们成功训练出一个(不含研发成本)计算费用低于 600 万美元的卓越模型。
3. Scaling up isn’t the only path to AI progress. For a long time, there has been enormous hype around scaling up models to drive AI progress. To be fair, I was once an early advocate for scaling up. Many companies raised billions by pitching the idea that more capital would allow them to (i) scale up and (ii) predictably drive improvements — thus overshading the fact that there are many other ways to advance AI. Partly due to the U.S. AI chip embargo, the DeepSeek team had to innovate and optimize in various areas so that their model could run efficiently on the less powerful H800 GPUs rather than on H100s, ultimately training a remarkable model for less than $6M in compute costs (excluding R&D expenses).
对算力需求的思考
这是否真的会减少对算力的需求,目前尚不得而知。事实上,有时降低单个单位的成本反而可能使总体支出增加。我认为,从长远来看,人类对智能和算力的需求几乎没有上限,因此即便智能变得越来越便宜,我们依然会利用更多的智能。
It remains to be seen whether this will actually reduce the demand for compute. Sometimes, lowering the cost per unit can lead to a higher overall expenditure. Over the long term, I believe that the demand for intelligence and compute is nearly limitless, so even as it becomes cheaper, humanity will continue to use more of it.
社交媒体上的多元解读与未来展望
我在社交媒体上看到人们对 DeepSeek 的进展有着各种解读,就像一个罗夏墨迹测试,每个人都能投射出自己的理解。我认为 DeepSeek-R1 的发布具有深远的地缘政治影响,这些影响还有待进一步观察与解读。对于 AI 应用开发者来说,这无疑是个令人振奋的消息。我的团队已经在集思广益,构思那些只有在我们能够轻松访问这些开源高级推理模型时才有可能实现的新想法。现在,依然是构建创新应用的黄金时代!
On social media, I’ve seen a variety of interpretations of DeepSeek’s progress — almost like a Rorschach test onto which everyone projects their own meaning. I believe that the release of DeepSeek-R1 carries profound geopolitical implications that are yet to fully unfold. For AI application builders, this is undoubtedly exciting news. My team has already been brainstorming ideas that are now possible thanks to our easy access to advanced open reasoning models. Truly, it remains a golden age for building innovative applications!
共勉,
Keep learning,
Andrew
最后附上这篇文章的原文链接:
https://www.deeplearning.ai/the-batch/issue-286/
我是木易,一个专注AI领域的技术产品经理,国内Top2本科+美国Top10 CS硕士。
相信AI是普通人的“外挂”,致力于分享AI全维度知识。这里有最新的AI科普、工具测评、效率秘籍与行业洞察。
欢迎关注“AI信息Gap”,用AI为你的未来加速。
(文:AI信息Gap)