
在人工智能的浪潮中,智能体技术正以其独特的能力成为研究与应用的新焦点。智能体,这些具备自主意识的数字精灵,能够敏锐感知环境、迅速做出决策并精准执行动作,为解决复杂问题提供了全新途径。从智能客服到自动驾驶,智能体的影响无处不在。
然而,传统的智能体开发框架往往效率低下,依赖特定模型或提供商,且对多模态数据支持不足。在这样的背景下,Agno应运而生,以其卓越的性能和灵活性,迅速在GitHub上获得了18.9K的Star,成为开发者的新宠。本文将深入探讨Agno这一开源项目,从项目概述、主要功能、技术原理、应用场景到快速使用,全方位解析,助您掌握这一强大工具。
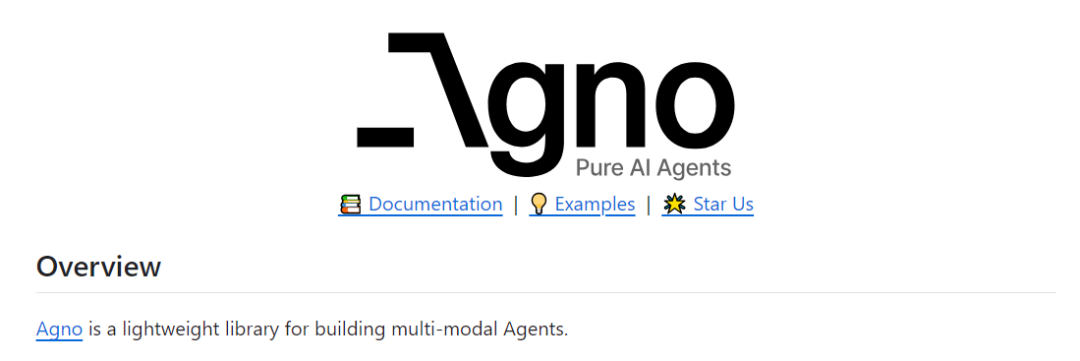
一、项目概述
Agno是一个专为构建多模态智能体而设计的轻量级Python库。它旨在为开发者提供一种简单、高效且灵活的方式来创建智能体,满足多样化的应用需求。
与传统框架相比,Agno的优势显著。在智能体创建速度上,Agno比LangGraph快约10000倍,极大缩短了开发周期。Agno的无依赖性架构,使其不依赖任何特定模型、提供商或模态,赋予开发者更大的选择自由。
二、主要功能
-
极速创建:Agno的智能体创建速度极快,平均实例化时间约2微秒,大幅提升开发效率。
-
模型无关性:支持任何模型和提供商,打破模型依赖,实现开发的灵活性和可持续性。
-
多模态支持:原生支持文本、图像、音频和视频,拓展智能体的应用领域。
-
多智能体协作:允许任务分配给多个专业化智能体,实现高效的分工和协作。
-
内存管理:将会话和状态存储在数据库中,提升交互体验。
-
知识库支持:利用向量数据库实现检索增强生成(RAG)或动态少样本学习。
-
结构化输出:支持以结构化数据格式响应,便于与其他系统集成。
-
实时监控:在agno.com平台上实时跟踪智能体会话和性能。
三、技术原理
-
简洁之美:基于Python编写,代码简洁易懂,易于上手。
-
灵活架构:无依赖性设计,适应不断变化的技术环境。
-
知识检索:集成向量数据库,提供强大的知识支持。
-
团队协作:多智能体协作机制,提高处理复杂任务的能力。
四、应用场景
Agno在智能客服、图像与视频分析、智能投资顾问、智能教育辅助等领域均有广泛应用,为各行各业带来智能化升级。
-
智能客服与聊天机器人:Agno可以帮助企业快速构建智能客服系统,利用其多模态支持和快速响应的特点,智能客服可以同时处理文本、语音等多种形式的客户咨询,快速准确地回答客户问题,提高客户满意度和服务效率。
-
智能图像与视频分析:Agno的多模态支持使得智能体能够对图像和视频进行深入分析,识别物体、行为、场景等信息。例如,在智能安防监控中,智能体可以实时分析监控视频,检测异常行为,并及时发出警报。
-
智能投资顾问:Agno的多智能体协作功能可以创建多个智能体,分别负责市场数据收集、数据分析、投资策略制定等任务。这些智能体协同工作,为投资者提供全面、准确的投资建议,帮助投资者做出更明智的投资决策。
-
智能教育辅助:Agno可以构建智能辅导系统,根据学生的学习情况和问题,提供个性化的学习建议和解答。例如,在在线学习平台中,智能辅导系统可以实时回答学生的问题,提供知识点讲解、作业批改等服务,实现24小时不间断的学习支持。
五、快速使用
1、安装:使用pip命令轻松安装Agno。
pip install-U agno
2、基本智能体示例:快速创建一个获取纽约突发新闻的智能体。
from agno.agent import Agent
from agno.models.openai import OpenAIChat
agent = Agent(
name="News Reporter",
role="Get breaking news stories",
model=OpenAIChat(id="gpt-4o"),
instructions="Only get stories from New York",
show_tool_calls=True,
markdown=True
)
agent.print_response("What's breaking news right now?", stream=True)
3、带工具的智能体示例:为智能体添加搜索功能,更准确地获取新闻信息。
from agno.agent import Agent
from agno.models.openai import OpenAIChat
from agno.tools.duckduckgo import DuckDuckGoTools
agent = Agent(
name="News Reporter",
role="Get breaking news stories",
model=OpenAIChat(id="gpt-4o"),
tools=(DuckDuckGoTools(),),
instructions="Only get stories from New York",
show_tool_calls=True,
markdown=True
)
agent.print_response("What's breaking news right now?", stream=True)
4、带知识库的智能体示例:结合向量数据库和知识源,创建专业的泰国美食专家智能体。
from agno.agent import Agent
from agno.models.openai import OpenAIChat
from agno.sources.pdf import PdfSource
from agno.vectorstores.lancedb import LanceDb
vector_store = LanceDb()
source = PdfSource("thai_cuisine.pdf")
vector_store.ingest(source)
agent = Agent(
name="Thai Cuisine Expert",
role="Answer questions about Thai cuisine",
model=OpenAIChat(id="gpt-4o"),
vector_store=vector_store,
instructions="Use information from the provided documents",
show_tool_calls=True,
markdown=True
)
agent.print_response("What are some traditional Thai cooking techniques?", stream=True)
5、多智能体团队示例:创建多个不同功能的智能体,组成团队处理复杂任务。
from agno.agent import Agent
from agno.models.openai import OpenAIChat
from agno.tools.duckduckgo import DuckDuckGoTools
from agno.tools.yfinance import YFinanceTools
web_agent = Agent(
name="Web Agent",
role="Search the web for information",
model=OpenAIChat(id="gpt-4o"),
tools=(DuckDuckGoTools(),),
instructions="Always include sources",
show_tool_calls=True,
markdown=True,
)
finance_agent = Agent(
name="Finance Agent",
role="Get financial data",
model=OpenAIChat(id="gpt-4o"),
tools=(YFinanceTools(stock_price=True, analyst_recommendations=True, company_info=True),),
instructions="Use tables to display data",
show_tool_calls=True,
markdown=True,
)
agent_team = Agent(
team=(web_agent, finance_agent),
model=OpenAIChat(id="gpt-4o"),
instructions=("Always include sources", "Use tables to display data"),
show_tool_calls=True,
markdown=True,
)
agent_team.print_response("What's the market outlook and financial performance of AI semiconductor companies?", stream=True)
结语
Agno作为一个轻量级的多模态智能体构建框架,以其快速、灵活、简单易用的特点,为智能体开发带来了革命性的突破。它不仅在功能上一骑绝尘,具有极快的智能体创建速度、模型无关性、多模态支持等显著优势,而且在技术原理上独具匠心,如纯Python实现、无依赖性架构等。通过本文的介绍,相信你对Agno已经有了更深入的了解。
无论是对于人工智能领域的资深研究者,还是对于希望将智能体技术应用于实际项目的开发者,Agno都无疑是一个强大的工具和平台。在未来,随着人工智能技术的持续飞速发展,相信Agno也将不断进化和完善,为智能体开发领域带来更多令人惊喜的创新,引领智能体开发进入一个全新的时代。
项目地址
(文:小兵的AI视界)