在多模态AI快速发展的今天,企业在数字化转型中面临着如何高效处理和理解复杂图像信息的挑战。
传统的RAG(Retrieval-Augmented Generation)系统主要依赖于文本数据,对于包含图像、图表、幻灯片等视觉信息的文档处理能力有限,常常需要将图像转换为Markdown格式,导致信息丢失和处理效率低下。
如今,借助Google DeepMind的Gemini 2.5视觉大模型和Cohere最新发布的Embed v4多模态嵌入模型,企业可以构建真正的视觉RAG系统,直接处理和理解复杂图像,跳过繁琐的图像到Markdown转换步骤,保留丰富的视觉信息,实现更高效、准确的多模态信息检索和问答。
一、传统RAG的局限性
传统的RAG系统在处理包含图像的文档时,通常需要将图像转换为Markdown格式,以便进行文本检索和生成。
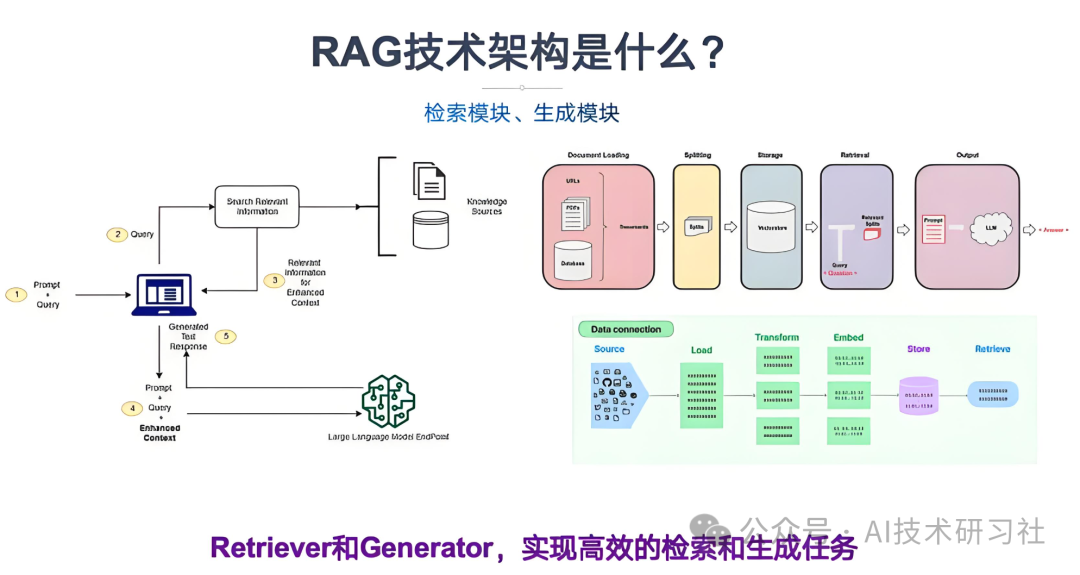
然而,这种方法存在以下问题:
-
信息丢失:图像中的细节和布局在转换过程中可能无法完整保留,影响理解和生成的准确性。
-
处理效率低:图像到Markdown的转换过程复杂,增加了系统的处理时间和资源消耗。
-
适应性差:对于多样化的图像类型(如幻灯片、图表、信息图等),传统方法难以统一处理。
二、视觉RAG的优势
结合Gemini 2.5和Cohere Embed v4的视觉RAG系统,能够直接处理和理解图像信息。
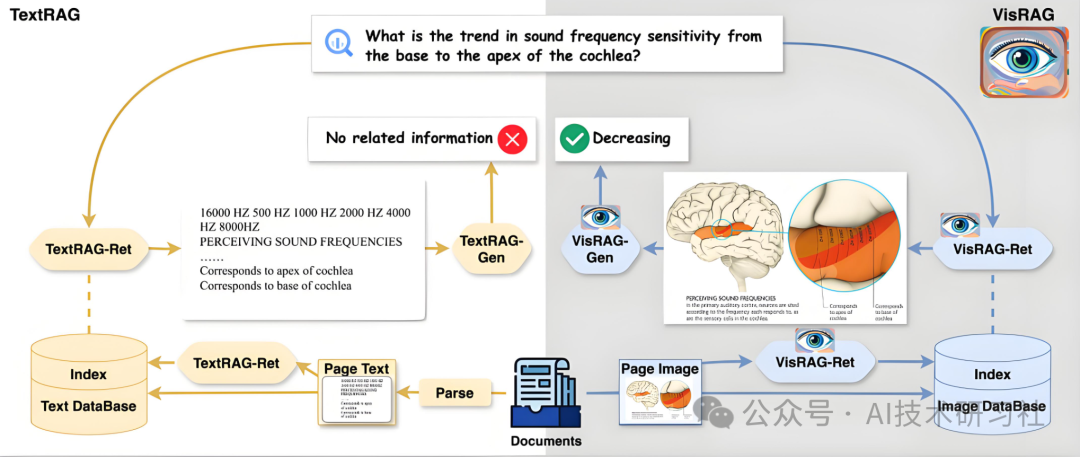
-
直接检索和理解复杂图像:无需转换,系统可以直接处理幻灯片、图表、图形、信息图等复杂图像,保留原始视觉信息。
-
提高问答准确性:通过推理复杂图形的内容,提供更准确、详细的答案。
-
增强多模态检索能力:Embed v4支持多语言和多模态数据的检索,适用于全球化企业的需求。
-
提升处理效率:跳过图像到Markdown的步骤,简化处理流程,节省时间和资源。
三、技术实现
1. Cohere Embed v4
Cohere Embed v4是最新的多模态嵌入模型。
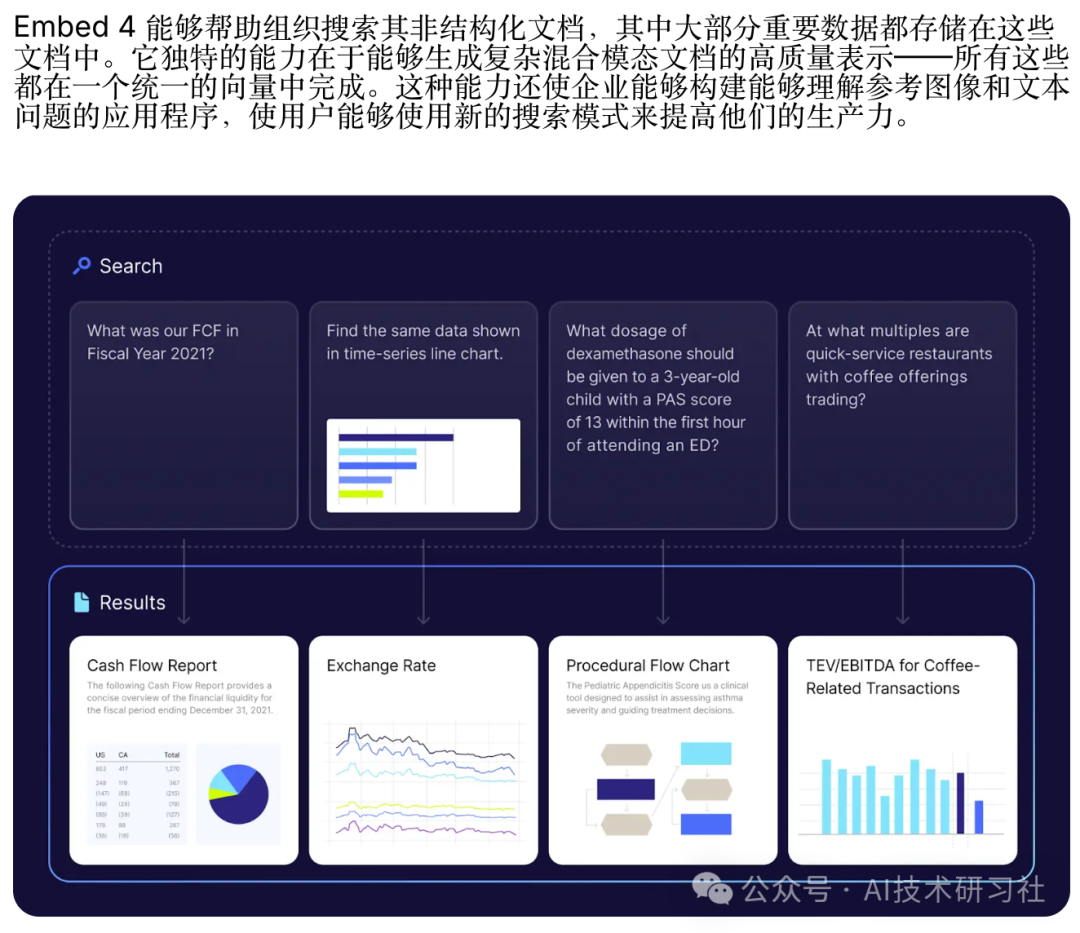
具备以下特性:
-
多模态支持:能够处理文本、图像、表格、图表等多种数据类型,生成统一的向量表示。
-
高准确性和效率:在复杂文档(如PDF报告、演示文稿)中实现快速、准确的搜索。
-
多语言能力:支持100多种语言,包括阿拉伯语、日语、韩语和法语,满足全球企业的需求。
-
增强的安全性:优化了对金融、医疗保健和制造等受监管行业数据的理解,支持虚拟专用云(VPC)和本地部署,确保数据安全。
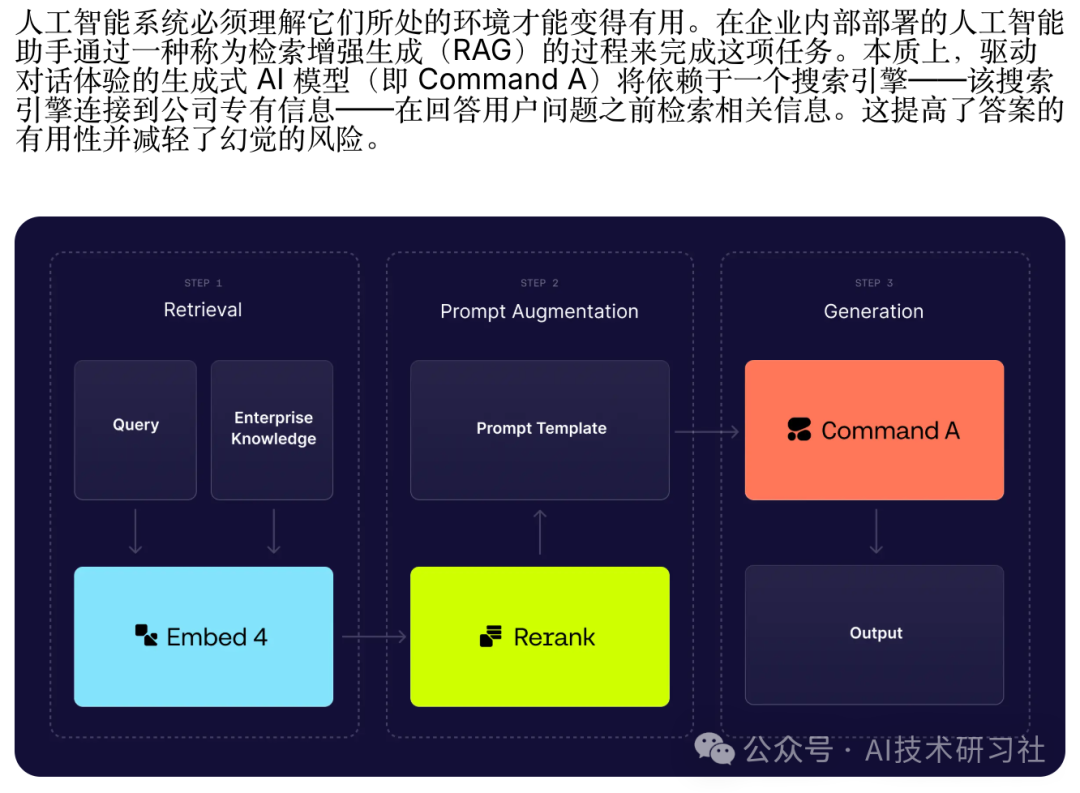
2. Gemini 2.5 Flash
Gemini 2.5 Flash是Google DeepMind推出的视觉大模型。
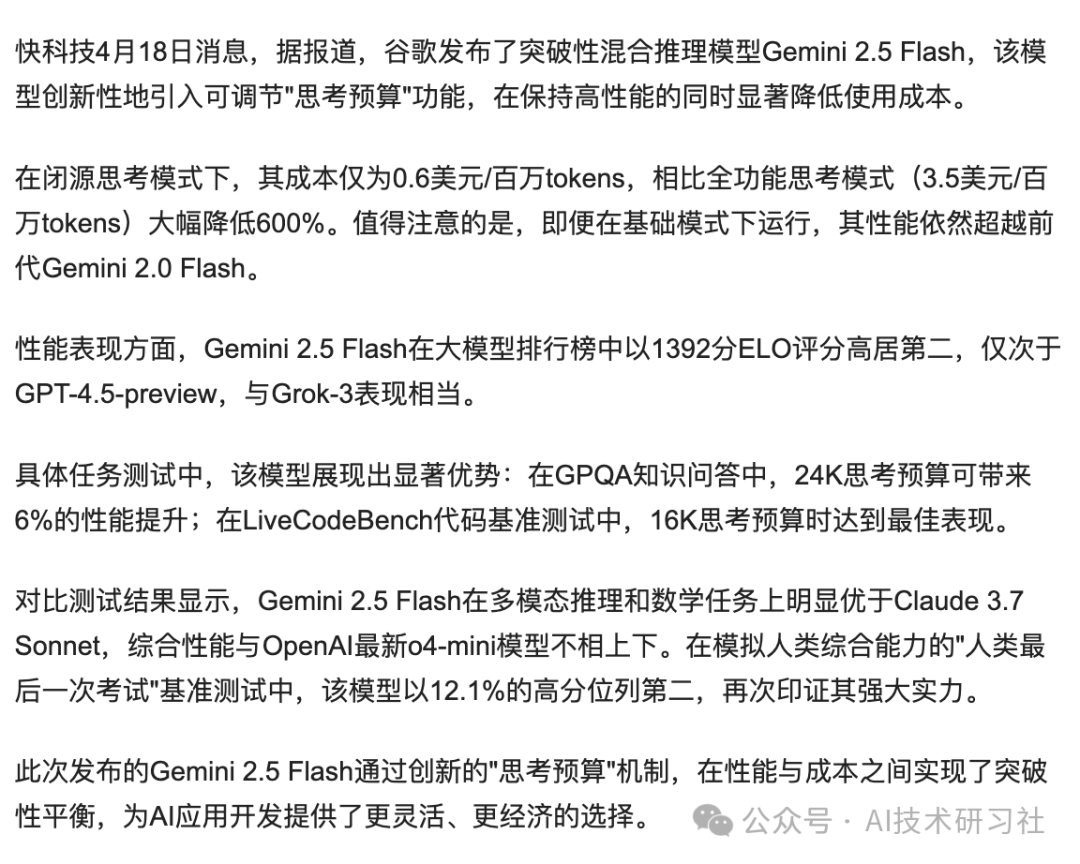
四、代码示例
到目前为止,RAG 主要在文本上完成。对于像 PDF 这样的丰富多媒体文件,通常需要复杂的 OCR 预处理步骤,并从您的 RAG 管道中删除相关图形。
以下是一个使用Cohere Embed v4和Gemini 2.5构建视觉RAG系统的简化示例。
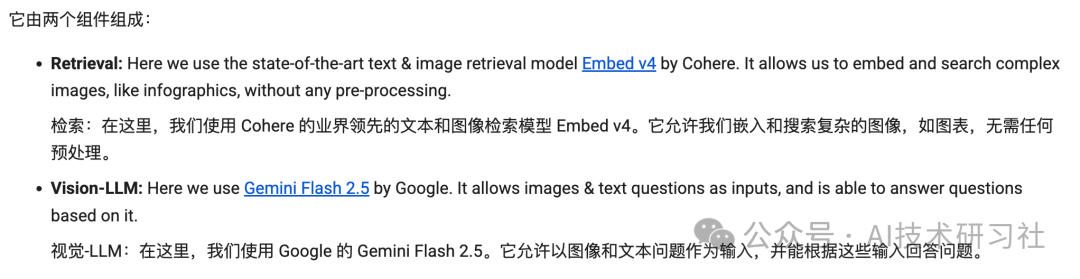
前往 cohere.com 获取 API 密钥。同时安装我们的 SDK 以简化使用,并安装 pdf2image 和 poppler 将 PDF 转换为图片。
pip install -q cohere
前往谷歌 AI Studio 生成 Gemini 的 API 密钥。Gemini 还提供慷慨的免费层。然后安装谷歌 GenAI SDK。
pip install -q google-genai
from google import genai
gemini_api_key = "<<YOUR_GEMINI_KEY>>" #Replace with your Gemini API key
client = genai.Client(api_key=gemini_api_key)
# Wrap long longs in this Notebook
from IPython.display import HTML, display
def set_css():
display(HTML('''
<style>
pre {
white-space: pre-wrap;
}
</style>
'''))
get_ipython().events.register('pre_run_cell', set_css)
本节将加载来自 appeconomyinsights.com 的多个信息图表。对于每张图片,我们将调用 Cohere Embed v4 来获取嵌入。这个嵌入允许我们稍后执行搜索,以找到与我们的问题相关的图片。
import requests
import os
import io
import base64
import PIL
import tqdm
import time
import numpy as np
# Some helper functions to resize images and to convert them to base64 format
max_pixels = 1568*1568 #Max resolution for images
# Resize too large images
def resize_image(pil_image):
org_width, org_height = pil_image.size
# Resize image if too large
if org_width * org_height > max_pixels:
scale_factor = (max_pixels / (org_width * org_height)) ** 0.5
new_width = int(org_width * scale_factor)
new_height = int(org_height * scale_factor)
pil_image.thumbnail((new_width, new_height))
# Convert images to a base64 string before sending it to the API
def base64_from_image(img_path):
pil_image = PIL.Image.open(img_path)
img_format = pil_image.format if pil_image.format else "PNG"
resize_image(pil_image)
with io.BytesIO() as img_buffer:
pil_image.save(img_buffer, format=img_format)
img_buffer.seek(0)
img_data = f"data:image/{img_format.lower()};base64,"+base64.b64encode(img_buffer.read()).decode("utf-8")
return img_data
# Several images from https://www.appeconomyinsights.com/
images = {
"tesla.png": "https://substackcdn.com/image/fetch/w_1456,c_limit,f_webp,q_auto:good,fl_progressive:steep/https%3A%2F%2Fsubstack-post-media.s3.amazonaws.com%2Fpublic%2Fimages%2Fbef936e6-3efa-43b3-88d7-7ec620cdb33b_2744x1539.png",
"netflix.png": "https://substackcdn.com/image/fetch/w_1456,c_limit,f_webp,q_auto:good,fl_progressive:steep/https%3A%2F%2Fsubstack-post-media.s3.amazonaws.com%2Fpublic%2Fimages%2F23bd84c9-5b62-4526-b467-3088e27e4193_2744x1539.png",
"nike.png": "https://substackcdn.com/image/fetch/w_1456,c_limit,f_webp,q_auto:good,fl_progressive:steep/https%3A%2F%2Fsubstack-post-media.s3.amazonaws.com%2Fpublic%2Fimages%2Fa5cd33ba-ae1a-42a8-a254-d85e690d9870_2741x1541.png",
"google.png": "https://substackcdn.com/image/fetch/f_auto,q_auto:good,fl_progressive:steep/https%3A%2F%2Fsubstack-post-media.s3.amazonaws.com%2Fpublic%2Fimages%2F395dd3b9-b38e-4d1f-91bc-d37b642ee920_2741x1541.png",
"accenture.png": "https://substackcdn.com/image/fetch/w_1456,c_limit,f_webp,q_auto:good,fl_progressive:steep/https%3A%2F%2Fsubstack-post-media.s3.amazonaws.com%2Fpublic%2Fimages%2F08b2227c-7dc8-49f7-b3c5-13cab5443ba6_2741x1541.png",
"tecent.png": "https://substackcdn.com/image/fetch/w_1456,c_limit,f_webp,q_auto:good,fl_progressive:steep/https%3A%2F%2Fsubstack-post-media.s3.amazonaws.com%2Fpublic%2Fimages%2F0ec8448c-c4d1-4aab-a8e9-2ddebe0c95fd_2741x1541.png"
}
# Download the images and compute an embedding for each image
img_folder = "img"
os.makedirs(img_folder, exist_ok=True)
img_paths = []
doc_embeddings = []
for name, url in tqdm.tqdm(images.items()):
img_path = os.path.join(img_folder, name)
img_paths.append(img_path)
# Download the image
if not os.path.exists(img_path):
response = requests.get(url)
response.raise_for_status()
with open(img_path, "wb") as fOut:
fOut.write(response.content)
# Get the base64 representation of the image
api_input_document = {
"content": [
{"type": "image", "image": base64_from_image(img_path)},
]
}
# Call the Embed v4.0 model with the image information
api_response = co.embed(
model="embed-v4.0",
input_type="search_document",
embedding_types=["float"],
inputs=[api_input_document],
)
# Append the embedding to our doc_embeddings list
emb = np.asarray(api_response.embeddings.float[0])
doc_embeddings.append(emb)
doc_embeddings = np.vstack(doc_embeddings)
print("\n\nEmbeddings shape:", doc_embeddings.shape)
以下展示了一个基于视觉的 RAG 的简单流程。
1) 我们首先执行 search() – 我们计算问题的嵌入表示。然后我们可以使用这个嵌入表示来搜索我们之前嵌入的图像,找到最相关的图像。我们返回这张图像。
2) 在 answer()函数中,我们将问题+图像发送到 Gemini,以获得我们问题的最终答案。
# Search allows us to find relevant images for a given question using Cohere Embed v4
def search(question, max_img_size=800):
# Compute the embedding for the query
api_response = co.embed(
model="embed-v4.0",
input_type="search_query",
embedding_types=["float"],
texts=[question],
)
query_emb = np.asarray(api_response.embeddings.float[0])
# Compute cosine similarities
cos_sim_scores = np.dot(query_emb, doc_embeddings.T)
# Get the most relevant image
top_idx = np.argmax(cos_sim_scores)
# Show the images
print("Question:", question)
hit_img_path = img_paths[top_idx]
print("Most relevant image:", hit_img_path)
image = PIL.Image.open(hit_img_path)
max_size = (max_img_size, max_img_size) # Adjust the size as needed
image.thumbnail(max_size)
display(image)
return hit_img_path
# Answer the question based on the information from the image
# Here we use Gemini 2.5 as powerful Vision-LLM
def answer(question, img_path):
prompt = [f"""Answer the question based on the following image.
Don't use markdown.
Please provide enough context for your answer.
Question: {question}""", PIL.Image.open(img_path)]
response = client.models.generate_content(
model="gemini-2.5-flash-preview-04-17",
contents=prompt
)
answer = response.text
print("LLM Answer:", answer)
# Define the query
question = "What is the net profit for Nike?"
# Search for the most relevant image
top_image_path = search(question)
# Use the image to answer the query
answer(question, top_image_path)
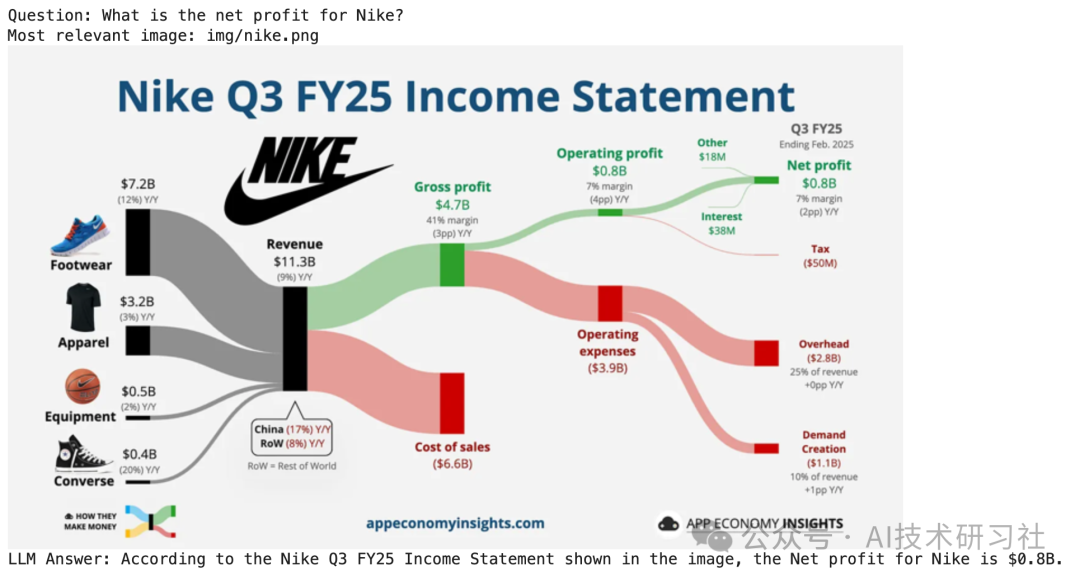
# Define the query
question = "What are the 3 largest acquisitions from Google?"
# Search for the most relevant image
top_image_path = search(question)
# Use the image to answer the query
answer(question, top_image_path)
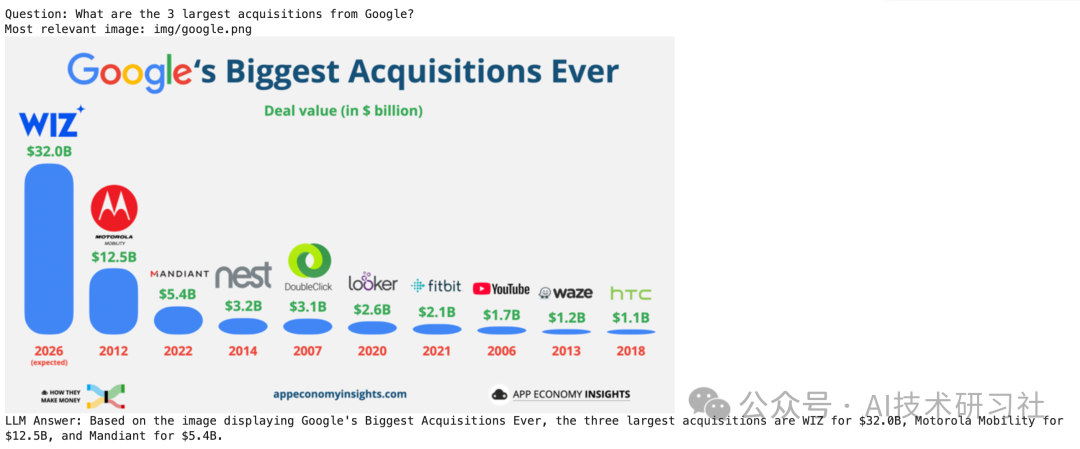
# Define the query
question = "What would be the net profit of Tesla without interest?"
# Search for the most relevant image
top_image_path = search(question)
# Use the image to answer the query
answer(question, top_image_path)
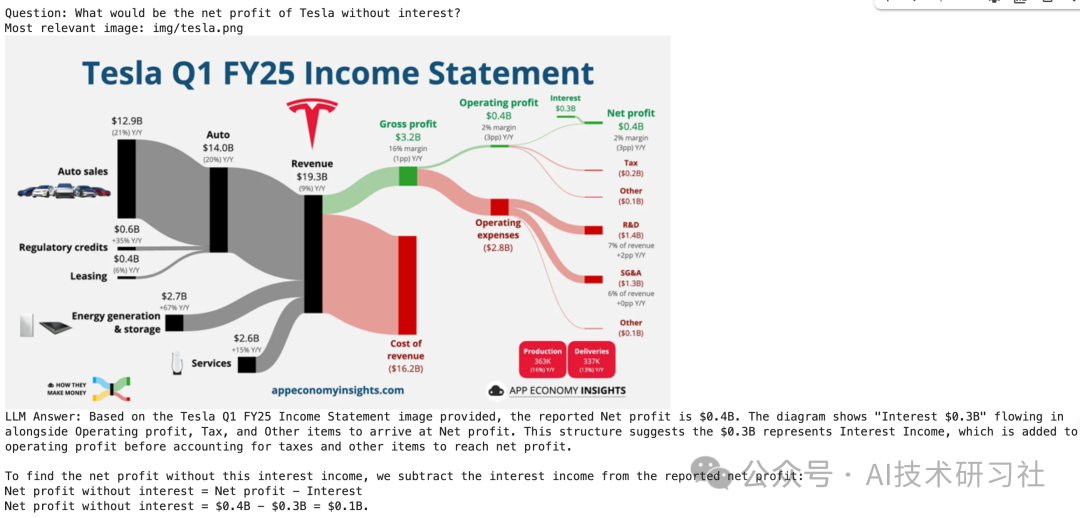
# Define the query
question = "Is GenAI a good business for consulting companies?"
# Search for the most relevant image
top_image_path = search(question)
# Use the image to answer the query
answer(question, top_image_path)
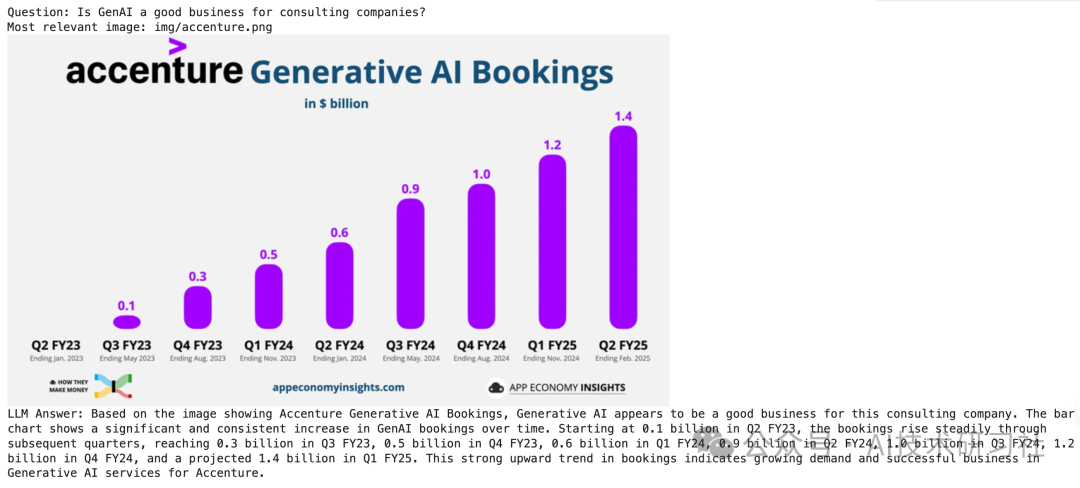
# Define the query
question = "In which region does Netflix generate the highest revenue?"
# Search for the most relevant image
top_image_path = search(question)
# Use the image to answer the query
answer(question, top_image_path)
# Define the query
question = "How much could tecent grow their revenue year-over-year for the last 5 years?"
# Search for the most relevant image
top_image_path = search(question)
# Use the image to answer the query
answer(question, top_image_path)
视觉RAG系统在多个行业中具有广泛的应用前景:
-
金融行业:分析投资者演示文稿、年度财务报告、并购尽职调查文件等,提取关键数据和洞察。
-
医疗保健:处理病历、流程图、临床试验报告等,辅助医生进行诊断和治疗决策。
-
制造业:理解产品规格文档、维修指南、供应链计划等,优化生产和维护流程。
-
教育领域:分析教学幻灯片、图表、信息图等,提升教学质量和学生理解能力。
通过将Gemini 2.5和Cohere Embed v4结合,构建的视觉RAG系统能够直接处理和理解复杂图像信息,跳过传统的图像到Markdown转换步骤,保留丰富的视觉信息,提高问答的准确性和效率,满足企业在多模态数据处理方面的需求。
随着多模态AI技术的不断发展,视觉RAG系统将在更多领域发挥重要作用,助力企业实现数字化转型,提升竞争力。
参考代码:https://colab.research.google.com/drive/1RdkYOTpx41WNLCA8BJoh3egQRMX8fpJZ#scrollTo=eUYg4r7JrDS2
(文:AI技术研习社)